Elevating user retention by 21%: Establishing trust in an AI SaaS education Web platform from 0-1
TIMELINE
Dec 2023 - Mar 2024
COMPANY
SmartPrep is an EduTech platform focused on transforming secondary education through AI-driven tools for language education.
ROLE & TEAM
Product Designer working with product manager and 5 software engineers.
RESPONSIBILITY
As the first design hire, I led user research to uncover user pain-points, built human-AI experiences from 0-1 to boost user retention, and established a design system for efficient handoff with cross-functional teams
Impact
+27%
teachers applied AI in teaching
-21%
churn rate across main user flow
$1.2M
secured seed round investment
Problem - User Focus
Teachers struggle to offer personalized support to students due to inefficient workflow.
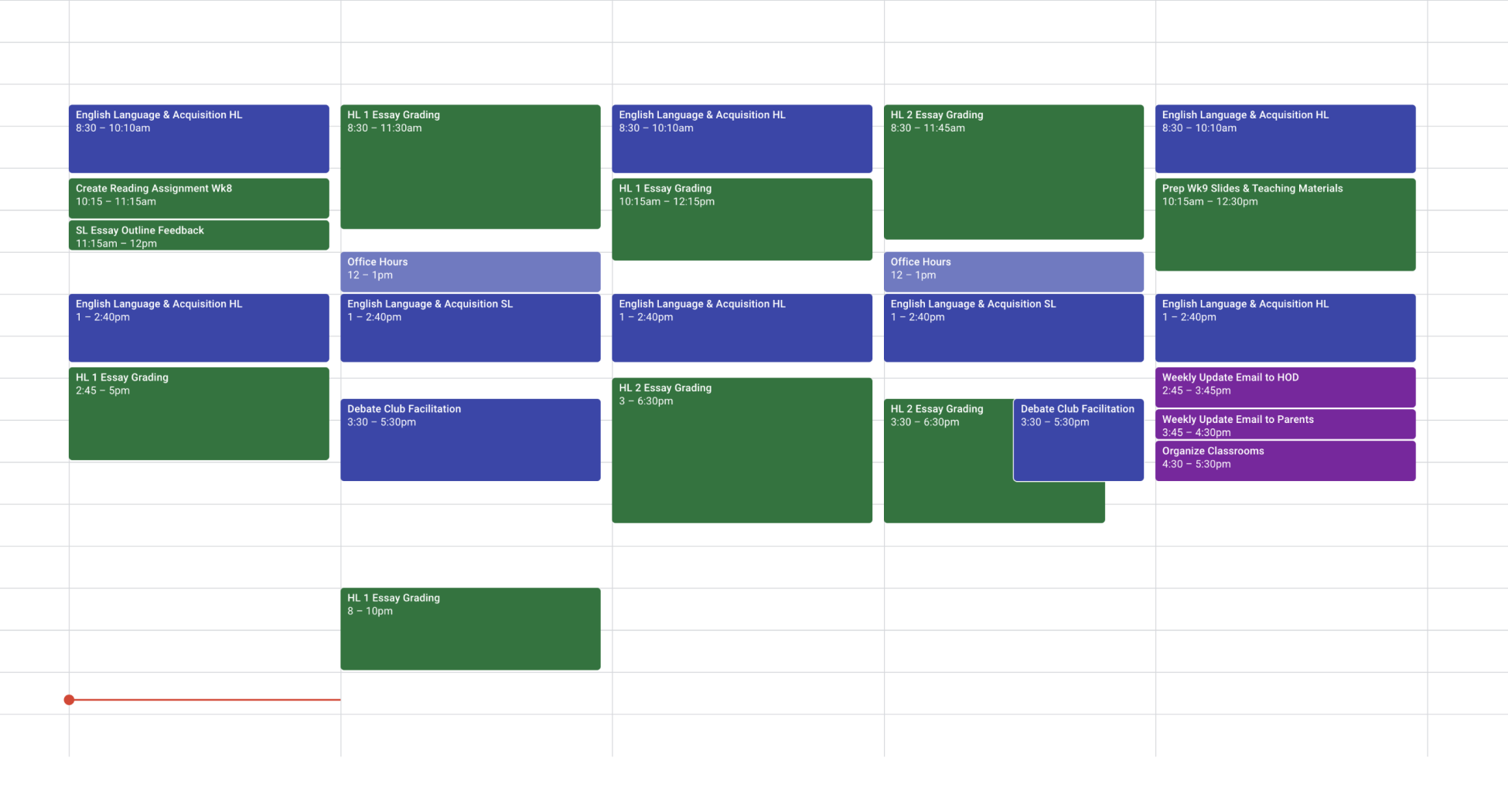
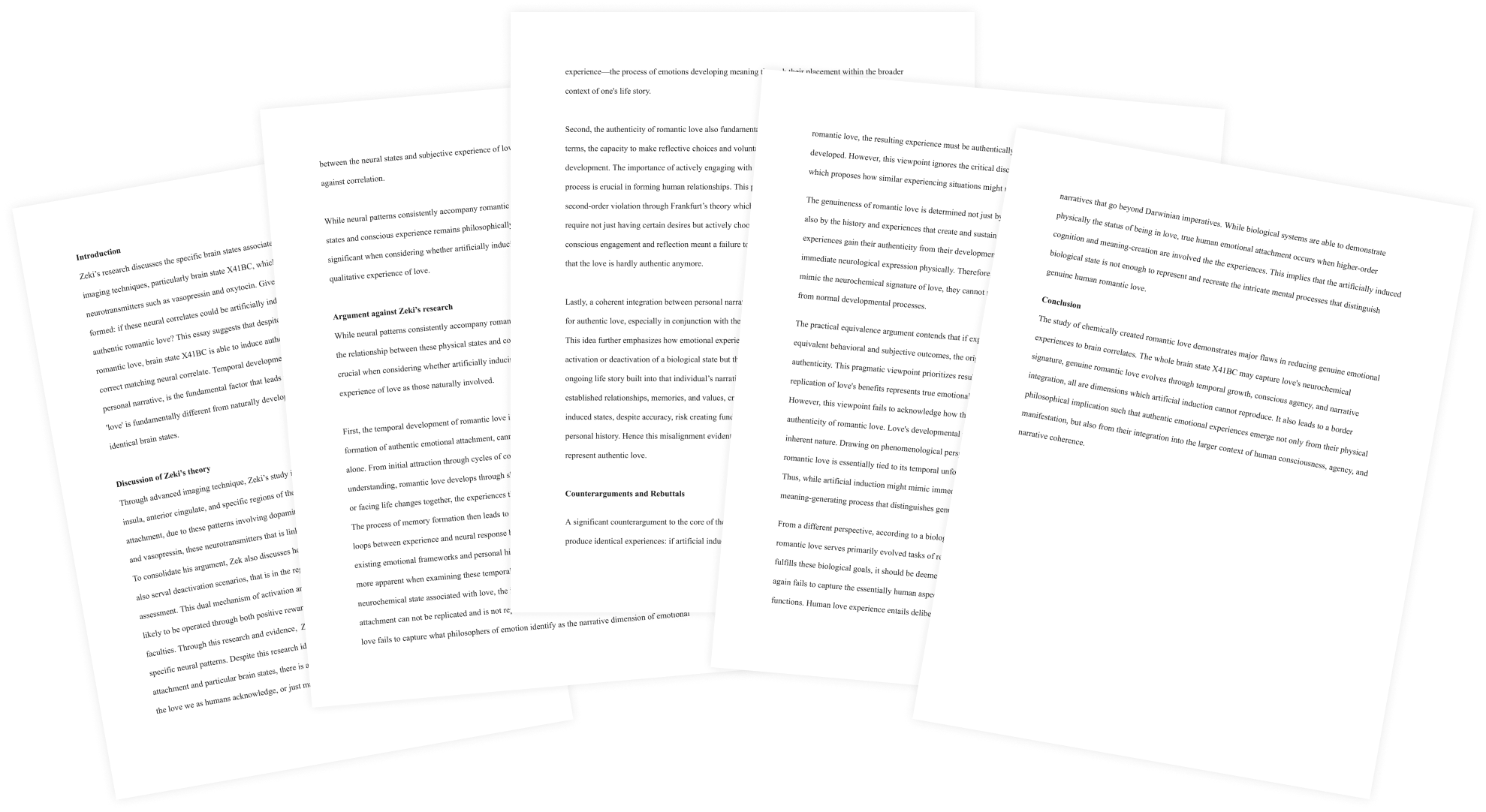
Teachers have little time for personalized suppport
Teachers are flooded with assignments to be graded
Problem - Business Focus
Low retention rate among trial users with our initial AI education chatbot attempt.
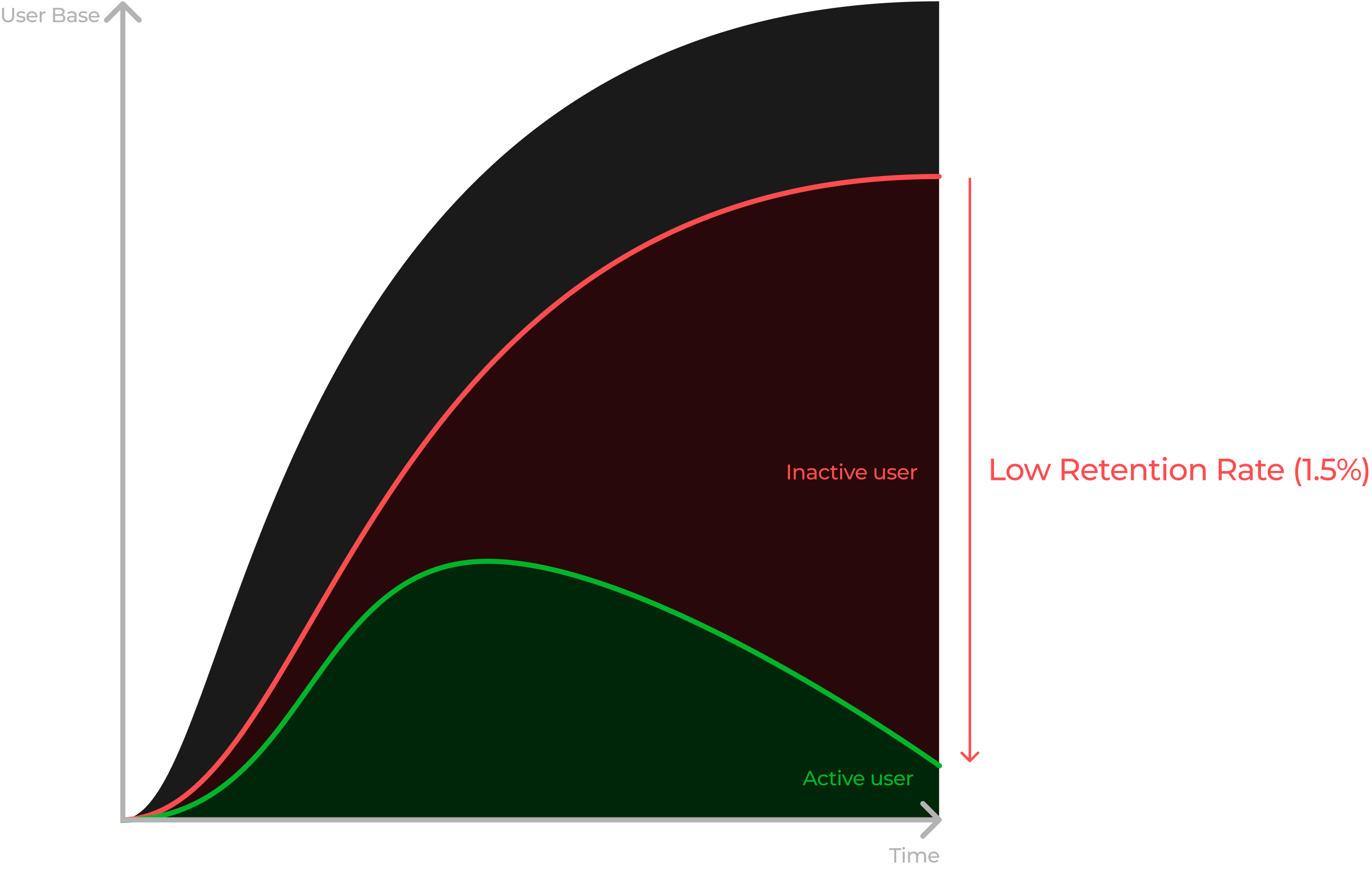
1.1 User Retention Graph
Solutions
A efficient and trustworthy education platform that supports teachers with personalized coaching.
Generate assignment with AI to help you save more time.
Teachers can generate entire assignments or specific questions using AI that aligns with their syllabus and learning objectives
2.1 Assignment Generation
2.2 Question Card Interaction
AI facilitated grading and student assignment analysis
Teachers can access information like AI grading suggestions, question breakdowns, common misconceptions, and more.
Personalized insight for every one of your student.
Over time, the AI will analyze student data, helping teachers uncover insights into strengths and weaknesses, and suggest areas for improvement.

2.4 Student Insights
So, how did I got here? It all started with...
Context -- Beta Product
Before I joined, due to time constrain the team started with a chatbot product.
Research -- Data ANalysis
96% of trial users dropped off before acting on AI generated insights.

4.1 User Engagement Map
Where... do we lost out user?
Mapping user retention across key touch points in the user journey to identify where we experienced the highest drop-off rates
Research -- Qualitative
Teachers are hesitant to rely on AI as there is too much uncertainty around the technology in education scenarios.
Why... do we lost our user?
To uncover factors behind low adoption and high drop-off rates, I cross-referenced interview notes from users and mocked a day in a life of teachers.
Iteration 1: User Flow
Teachers have a complex role and prefer products that support the entire workflow.
However, the chatbot only covers limited workflow.
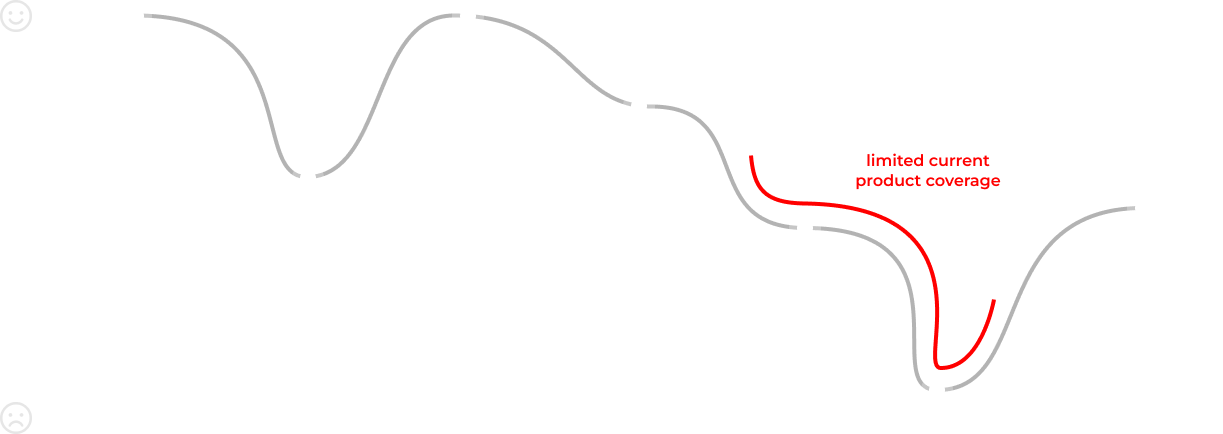
User Workflow and Curret Product Coverage
Expanding product coverage
Users were accustomed to using different platforms for assignments. Hence, we expanded our product to mimic their familiar workflow.
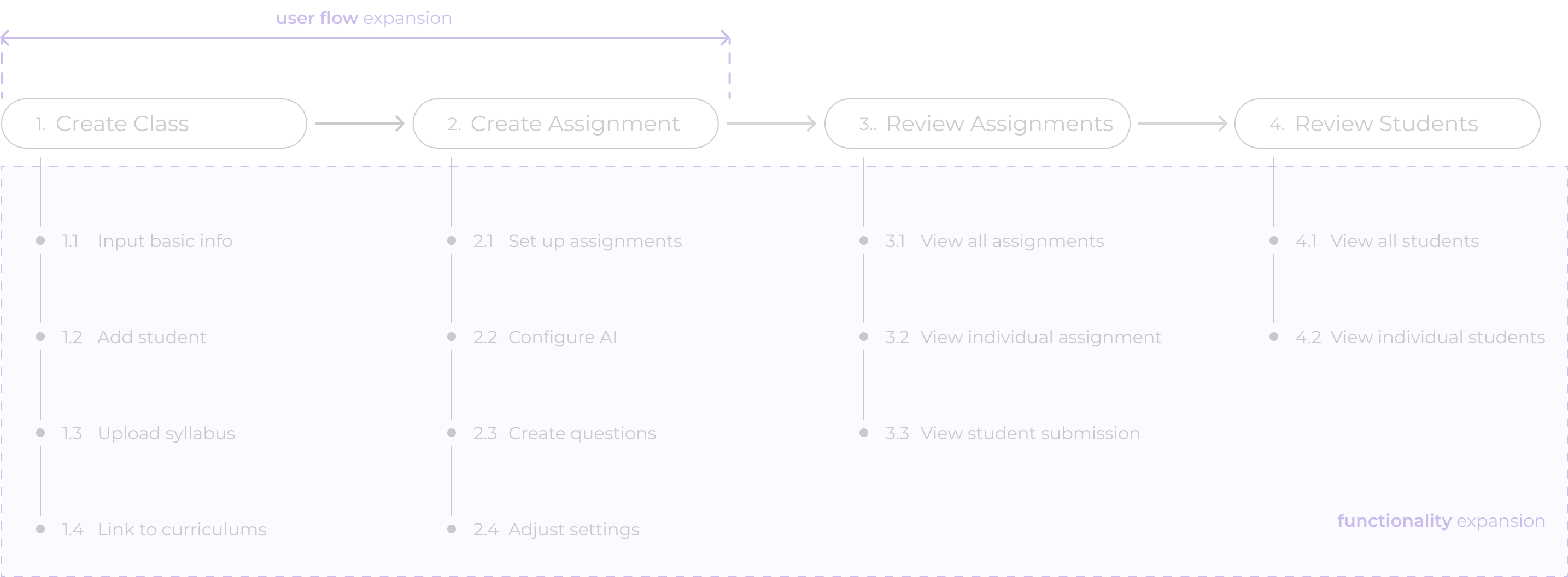
User Workflow and Curret Product Coverage
Unresolved Problem
Low user trust is the root cause of product abandonment, reducing product retention.
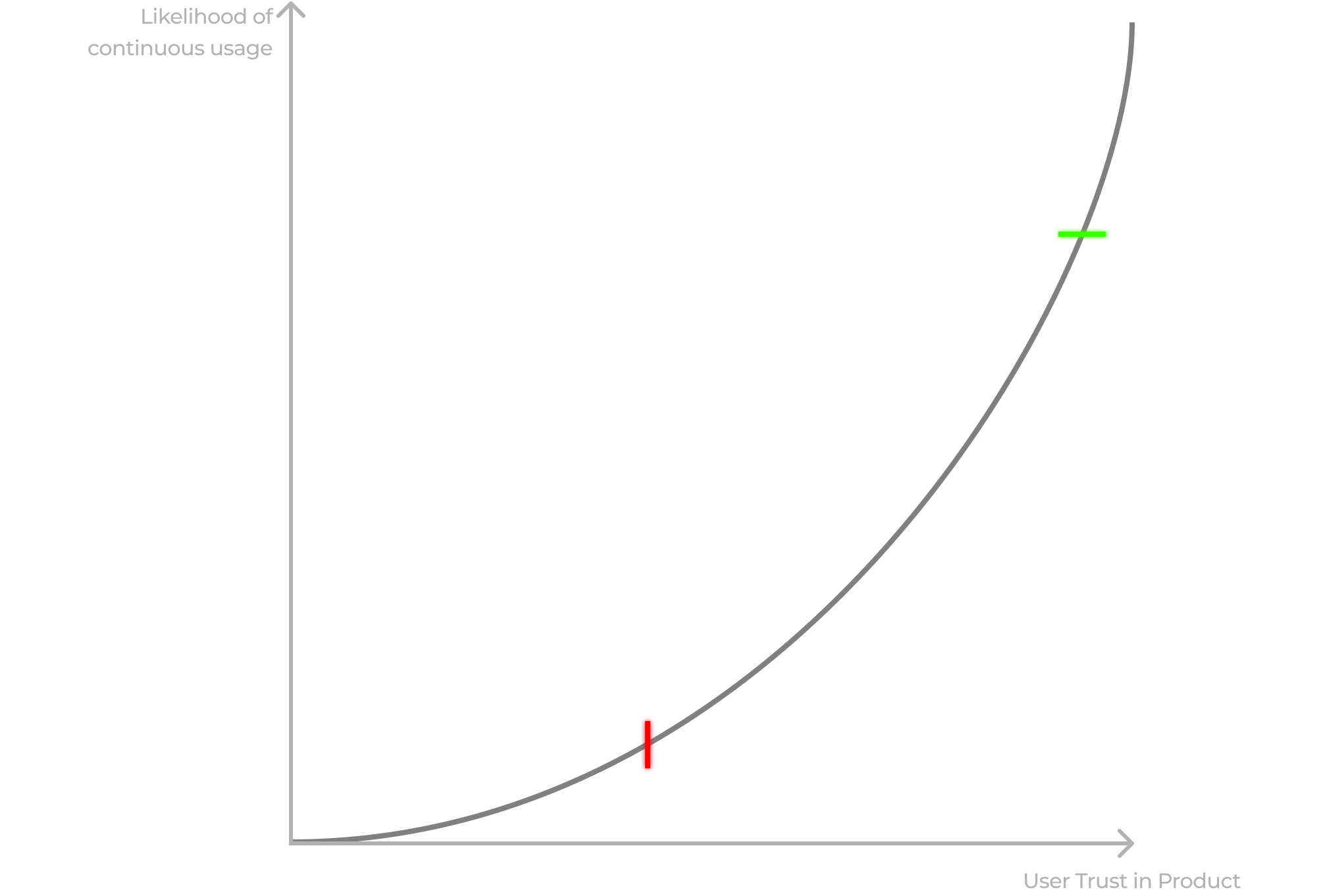
1.2 User Trust & Continuous Usage Rate
Enhance the credibility of AI interactions to further reduce uncertainty and build trust.
User's trust emerges from repetitive reliability indicator, but the question is how ...?
To explore ways to strengthen trust between users and AI products, I delved into HCI research on AI interactions scenarios that demands high level of user trust, and examined the concept of reliability indicators that justifies trustworthiness.
Design Principles for AI interactions
AI in education should provide familiarity and transparency for teachers
Design the interface and interactions to align with existing educational tools, reducing the learning curve and making the AI feel more approachable.
Clearly entail how AI makes decisions and where AI is implemented, providing users with understandable explanations and insights into the processes.
Iteration 2: AI Interaction in assignment Creation
Helping teachers feel more comfortable with AI via using familiar prompt inputs.
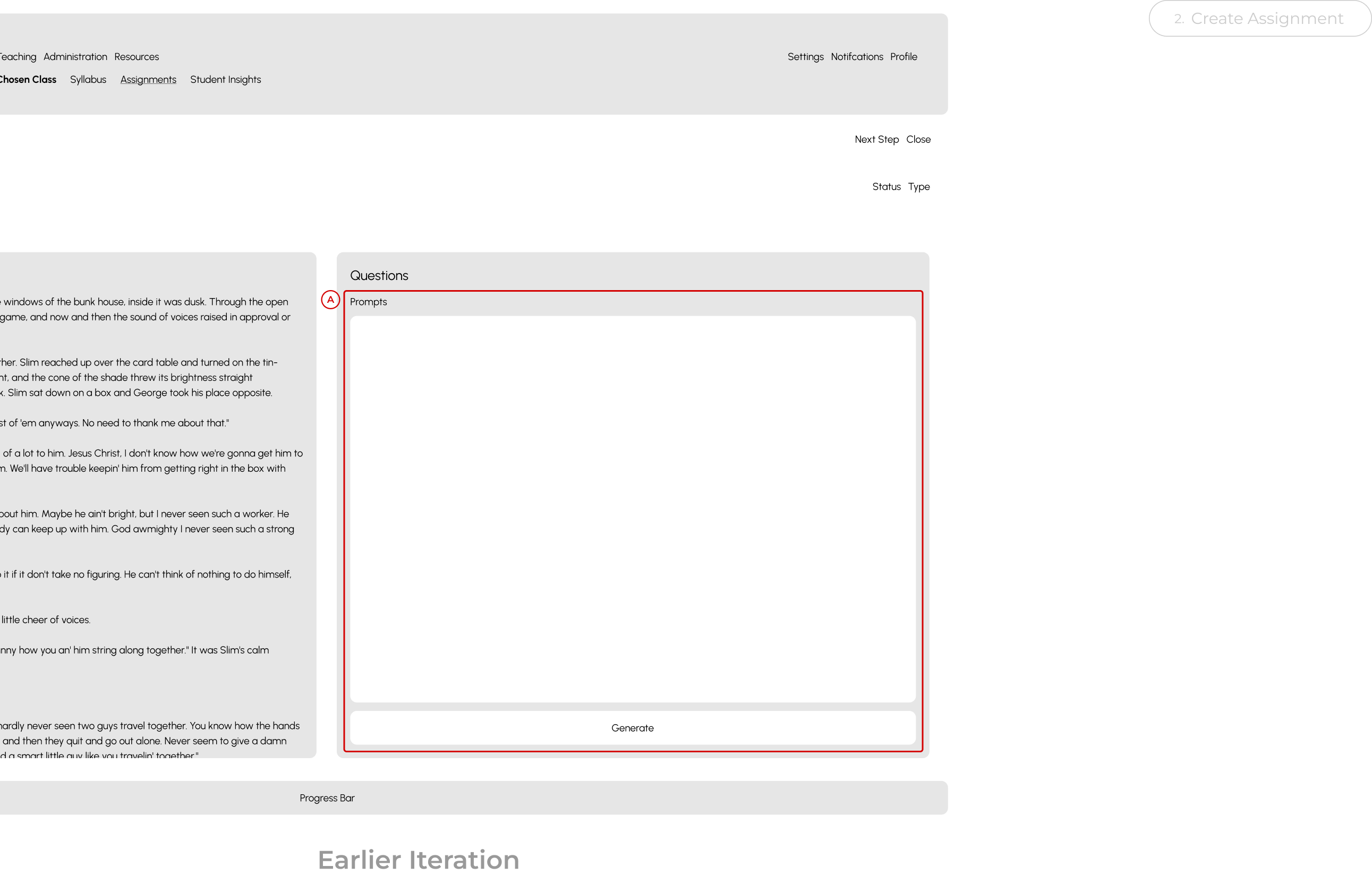

Ensure clarity in potential AI interactions to empower users with autonomy and eliminate uncertainty.
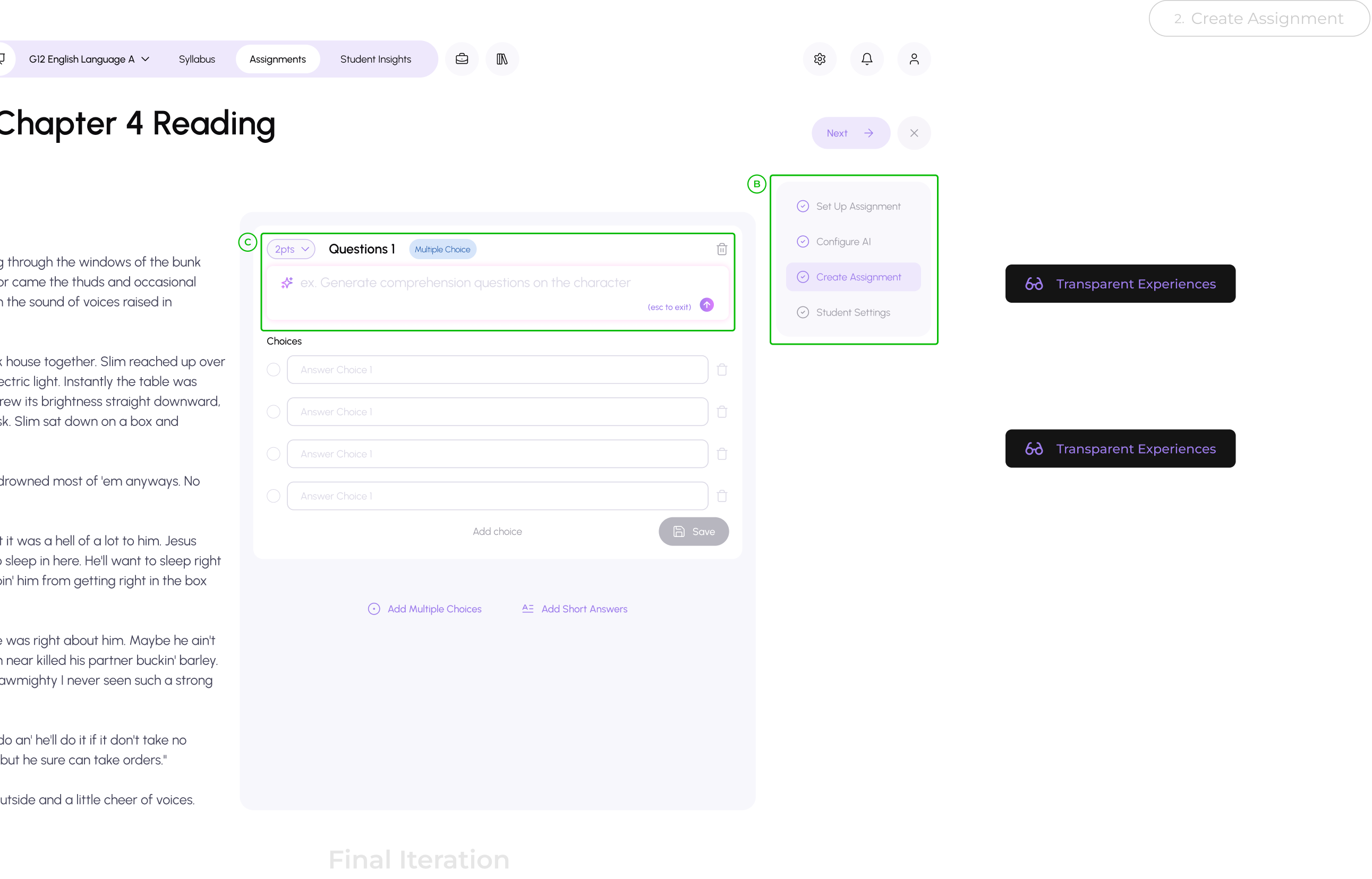
Iteration 2: AI Interaction in assignment Grading & Analysis
Transparency with AI involvement to help teachers make better judgement themselves.
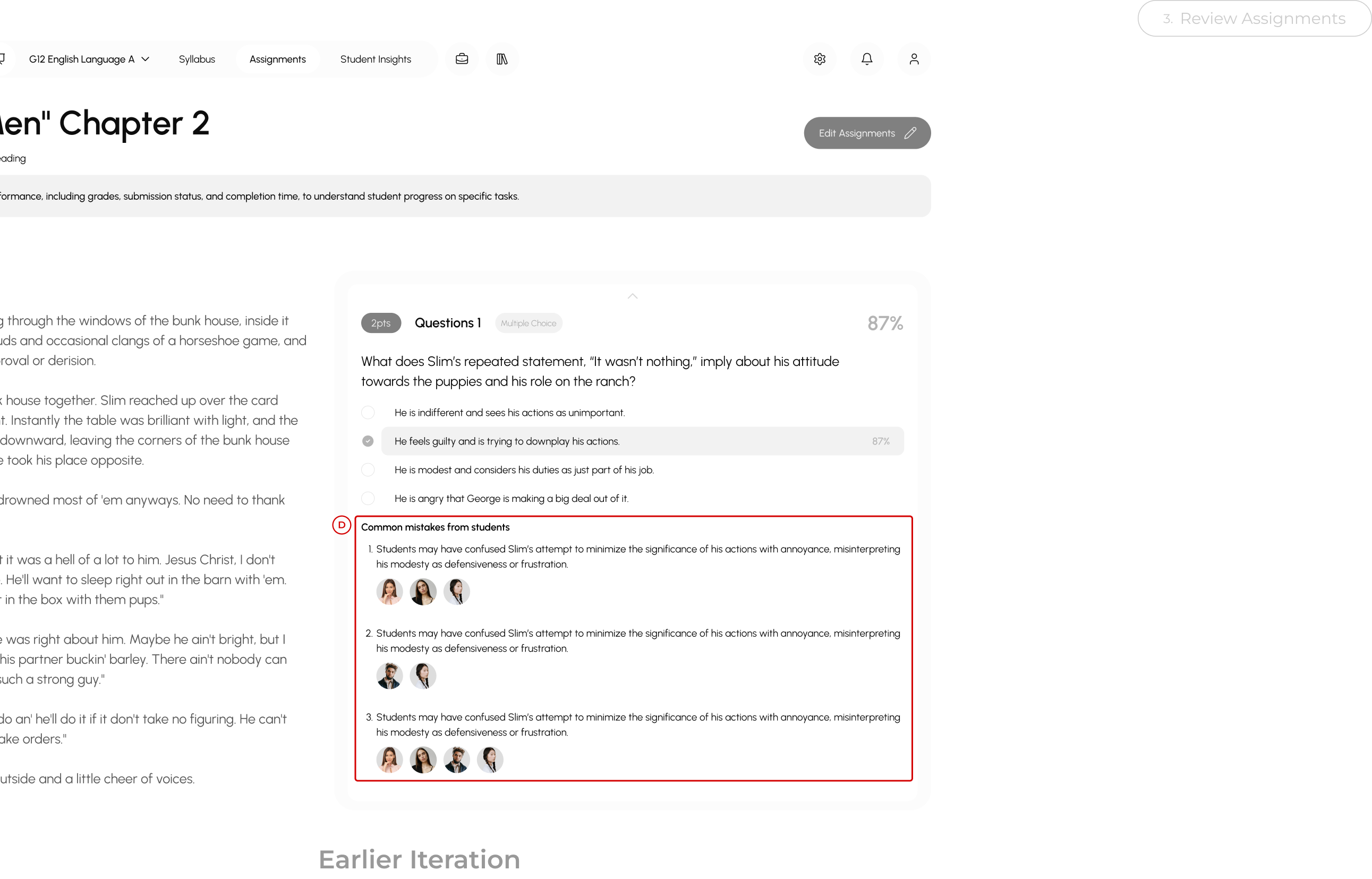
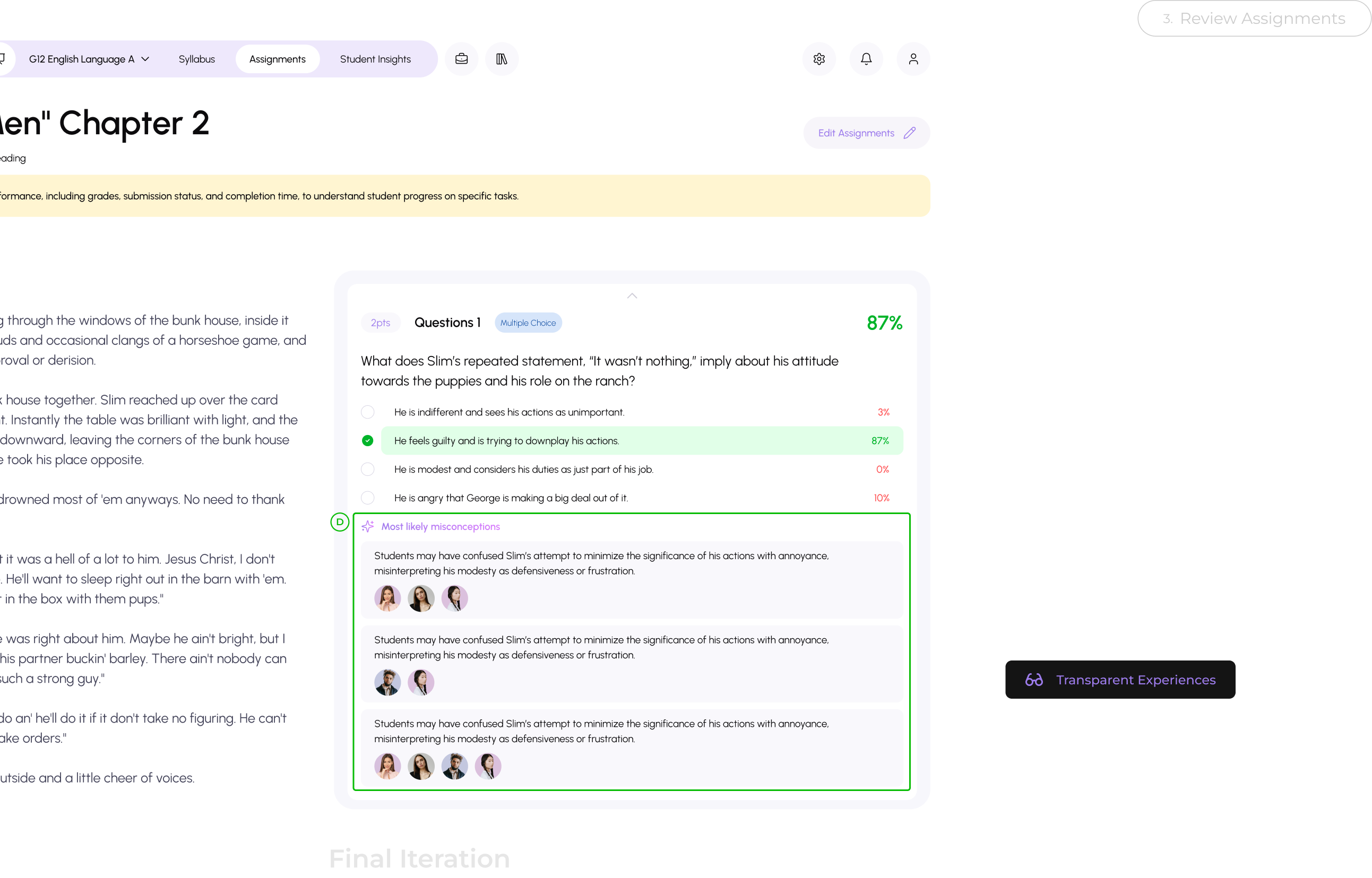
Transparency in students’ interactions with AI fosters trust between platforms and teachers.
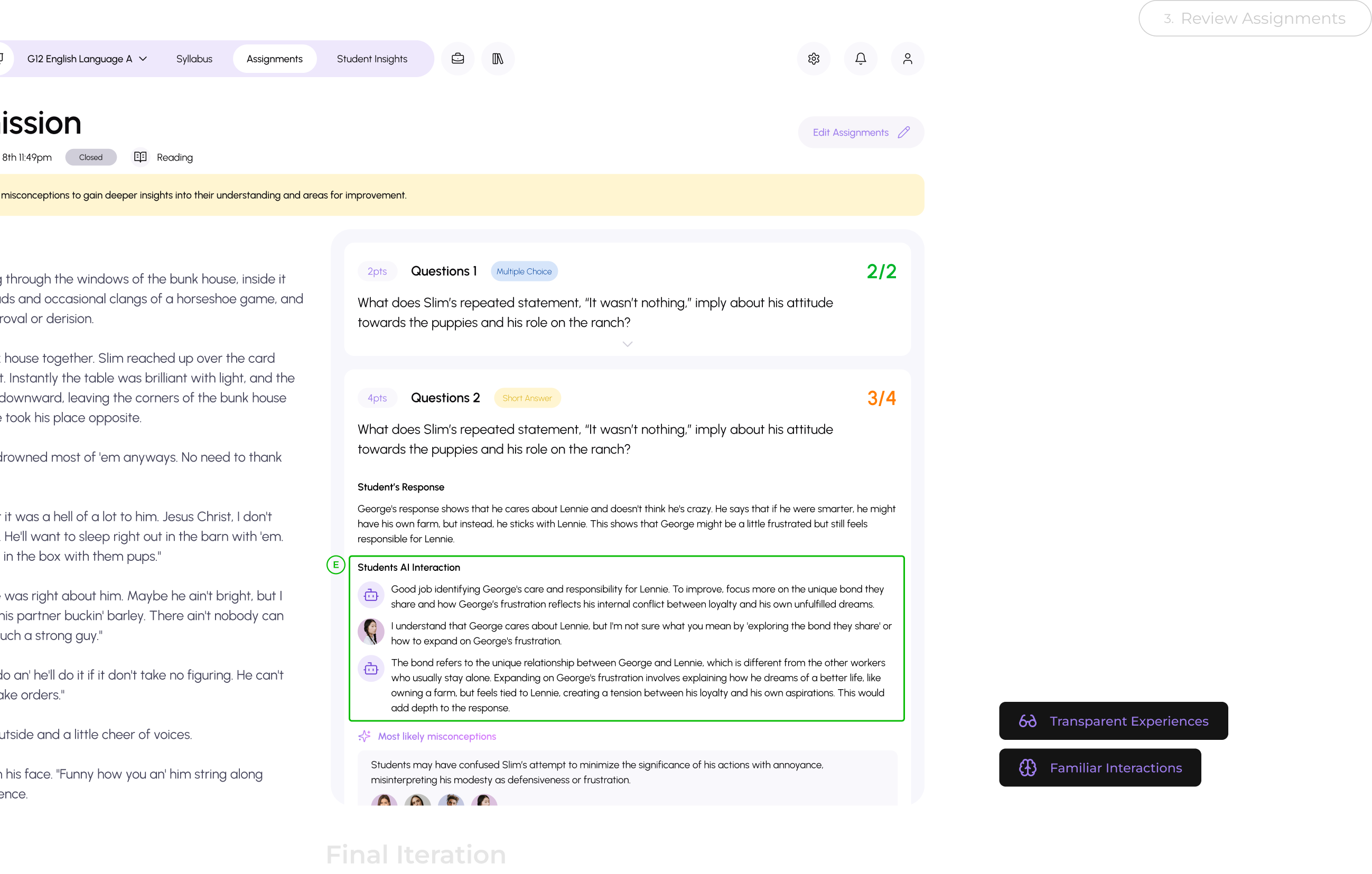
Iteration 2: AI Interaction in Student Insights
Transparency with evidence and explanation to calibrate trust in the moment.
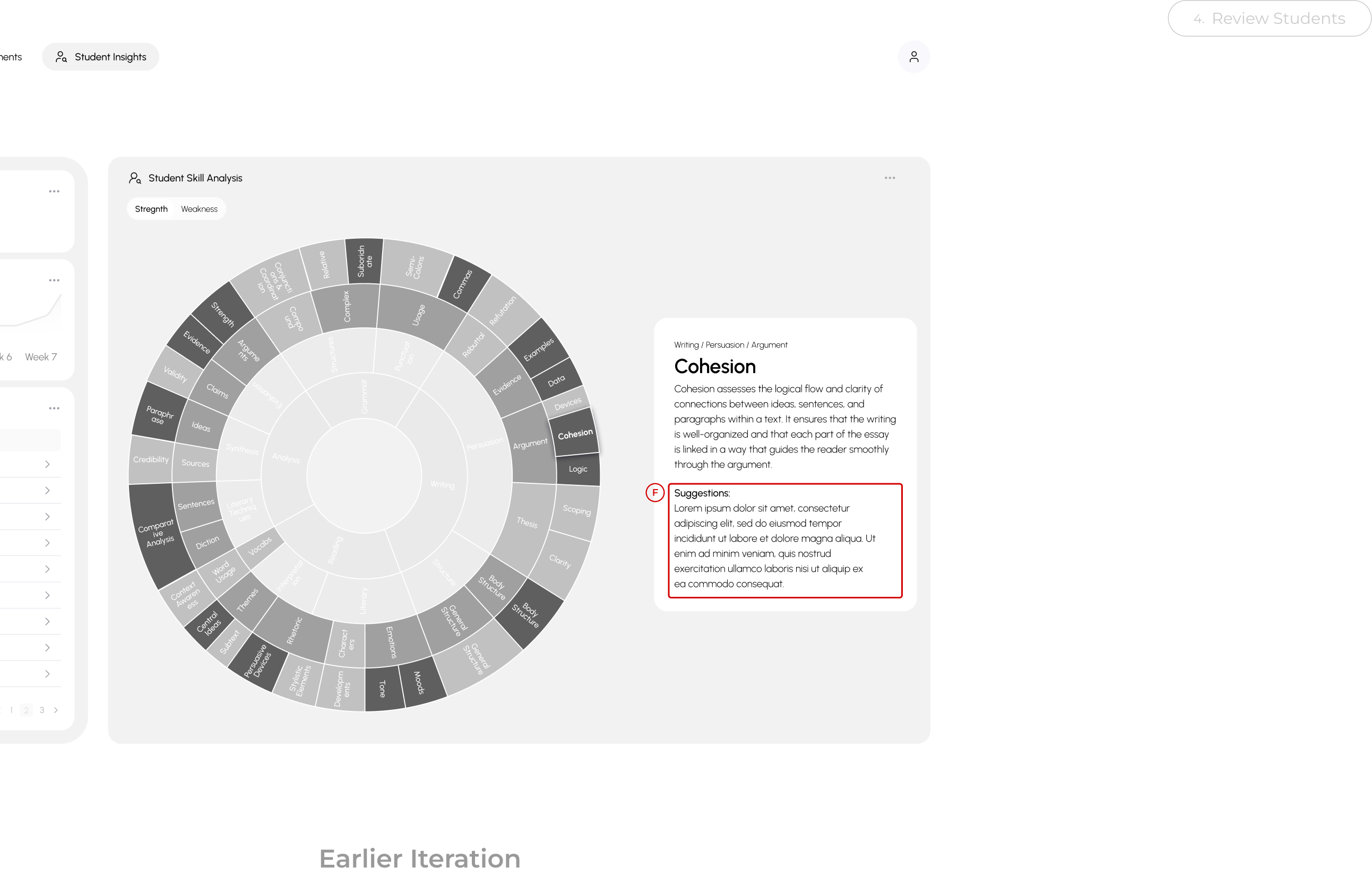
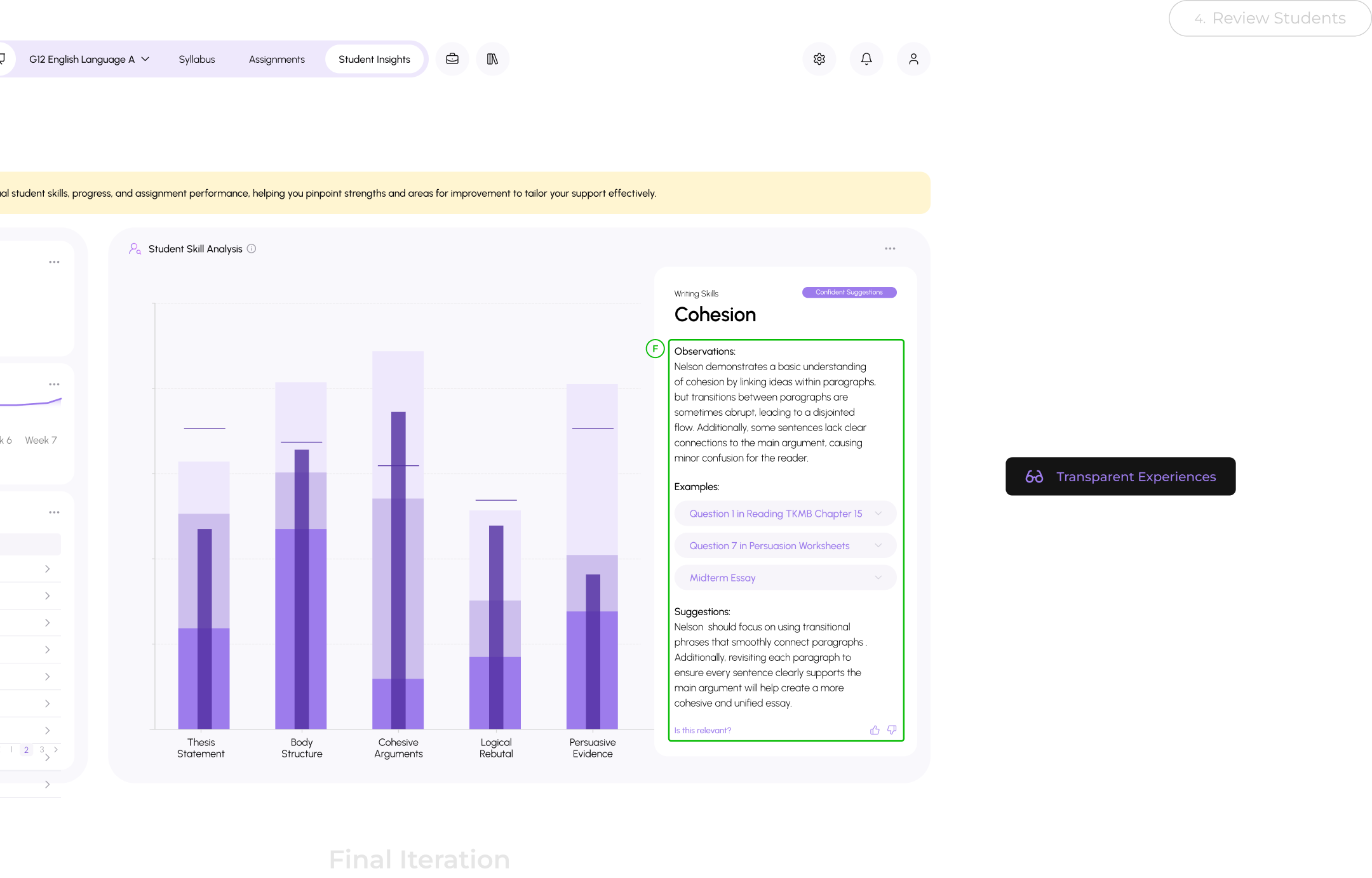
Transparency with confidence level indicator to be honest with our users.
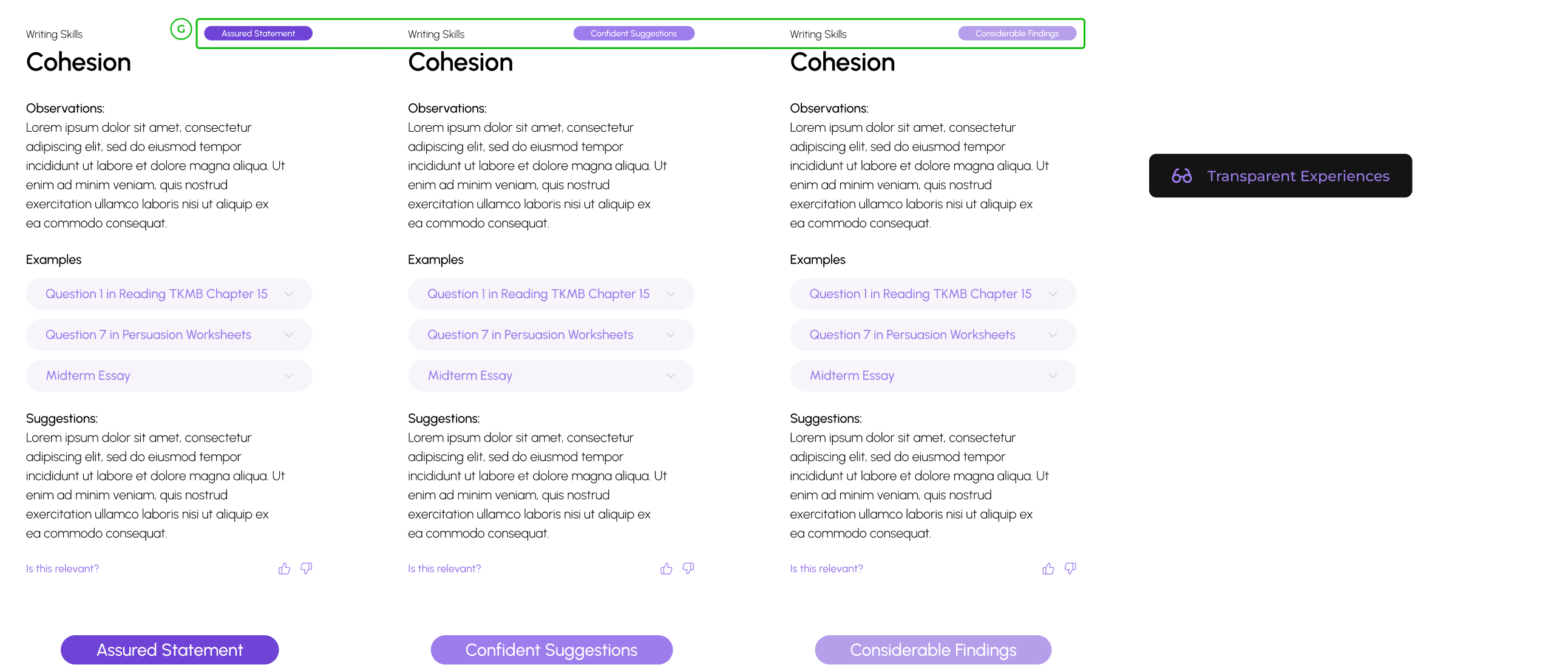
View the final solutions again ↑Retrospectives
Designing for Implementation Means Designing with Engineers in Mind
In a fast-paced startup environment, I learned that collaborating closely with engineers is crucial. From mapping out all edge cases to providing clear annotations and structured handoffs, making life easier for engineers means more of my designs get pushed out to users. Seeing my Figma work come to life is incredibly rewarding.
Navigating Ambiguity in Emerging Tech with Academic Research
Designing for AI comes with a lot of ambiguity due to the lack of established design patterns. Instead of relying on intuition alone, I found that academic research in HCI, especially on human-AI interaction, provided valuable guidance in shaping the cognitive models of AI interactions.
Prioritizing Designs that Drive Business Growth
At an early-stage startup, I had to prioritize designs that would have the most impact on business growth. Engaging closely with the business side, I realized that launching a functional version—even with minor design flaws—can be crucial for meeting client timelines, generating revenue, and gathering data to improve the product.